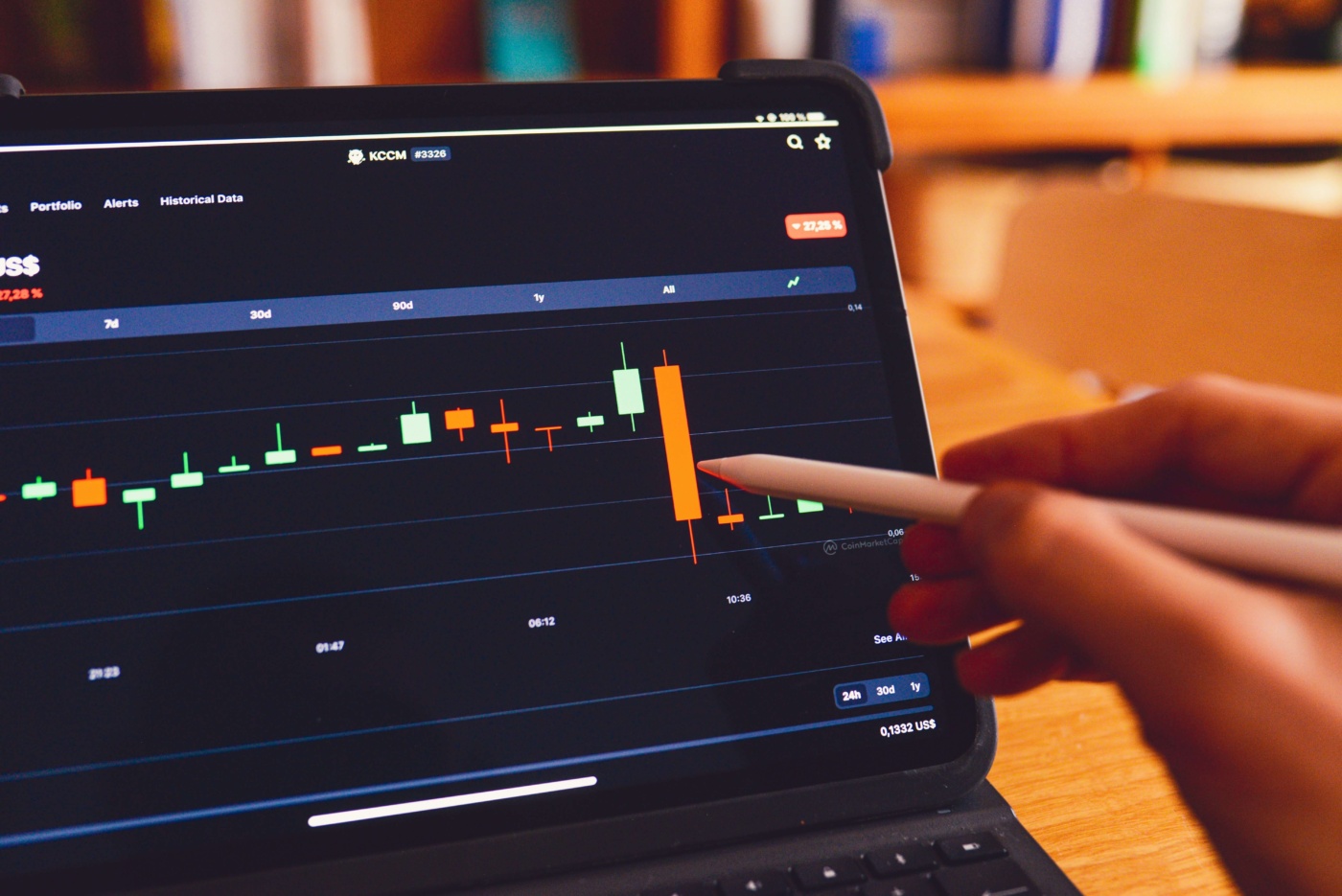
Industry Spotlight: Quantative Analysis
Introduction
Quantitative Analysis. It's a topic that little of us know much about. Unless you work in hedge funds or you're a die hard fan of the hit TV series Billions, it is unlikely you will have heard much about this industry.
The quant fund industry is really important. It is shaping our future and becoming increasingly more relevant as we, as a global society, question what our data is being used for.
Quantitative analysis shapes capital markets. It addresses questions and data collected from pretty much every data source in every market imaginable and answers some of the biggest questions in finance.
As the connectivity provider for some of the leading financial and quantitative analysis organisations in the world, we thought we would put a spotlight on the industry and provide some perspective on what it does, and why content specialist connectivity is critical.
What is Quantitative Analysis?
Quant analysis, by and large, underpins quant funds. Quant funds are investment funds where securities are selected using numerical and statistical methods which are compiled through quant analysis rather than human analysis – as is done in traditional hedge funds. If you are a Billions fan, you may recount the main character who owns a hedge fund claim that a decline in returns on his hedge fund was due to a rise in the number of quant funds.
So, think of quant analysis and quant funds as one and the same – they are loosely referred to as the same thing. The vital part here is that it is quantitative analysis that makes trading decisions.
Quants (the term used to describe people that work in quant analysis) come up with a hypothesis, test it through their various technologies using big data, model it, and then provide that data to traders to place trades on that information. Simple, right?
Using Big Data to Predict the Future
Broadly, this industry predicts what will happen tomorrow to the financial markets. It looks at the past to predict the future.
Using advanced mathematical modelling, statistical analysis and cuttingedge software, an expansive set of data from countless sources is intensely analysed. Deep insights are made and patterns are found in large, noisy and real-world data sets where you would not imagine an important correlation to exist. They might seem really random. Such as, how many more people buy coffee on a sunny day compared to cloudy? Are more people using bikes? If so, why, how and where? What does this affect?
Such analysis allows for identification of profitable trading opportunities. These predictions are then sent to (trading houses e.g. investment institutions, hedge funds) trading sites across the globe.
The Industry Connectivity and Quant Funds
So, now we know what quant funds do, what their objectives are, and broadly how they work, we can look at another crucial stage: Transportation.
Transportation of their expansive data sets, and transportation of their predictions and judgements to trading sites. Let's break this down.
How do the never ending data sets reach quants in the first place? How can they successfully pull volumes of content with speed, reliability and volume? With high capacity connectivity, of course.
As we all know, moving data around is the lifeblood of most industries today. However, moving BIG DATA (in every sense) requires that extra reliance on high bandwidth connectivity. In addition, the point at which this data is computed – i.e. run through supercomputers to complete their mathematical modelling, programming and analysis, would not happen without the correct connectivity powering it.
Do people feel more pain on rainy days?
This thinking is an example of illusory correlation, a type of confirmation bias in decision making. Searching for information or interpreting information in a way that supports our preconception can lead to a biased outcome.
Experiments designed to prove (not disprove) a theory can result in erroneous conclusions about the accuracy of the theory. In the example above, considering the situations where the relationship between pain and rain does not exist is equally relevant to determining the correlation between the events.
This example demonstrates the possibilities in quant analysis – a seemingly random and unimportant hypothesis is analysed to tell them something, whether it is good or bad. Apply this on a global scale, and you can see why big data and machine learning is a vital part of this industry. In order to arrive at new-found predictions, a ginormous volume of data, questions and hypotheses need to be tested.
What Happens to Those Decisions?
The objective decisions are then utilised and applied in many different ways. In this case, they are used for executing trades. This means they must reach the trading centres – which use the decisions to execute trades – as quickly as possible, so that other data judgements are not used from competing sources that arrive in on normal time. As such, the connection in which these decisions are transported from the quants' offices to the trading centres, must be ultralow latency.
So, high capacity point-to-point connections deal with the volume of data in use. Low latency enables their output to travel as quickly as possible to make all the hard work worthwhile.
Compiling intelligent connectivity solutions that accommodate these requirements is our speciality, it's how we support organisations in this industry to do what they do best. However, there is one final piece of the connectivity puzzle we have not mentioned, and that is equally as critical for quant funds: uptime and resilience.
Our Turn to Predict the Future
Normally, from a traditional carrier, organisations would have their point-to-point services built as Ethernet protected by their carriers' MPLS backbone. This achieves a high degree of resilience and redundancy, and also benefits from the performance monitoring and other operations and management capabilities that Ethernet brings over lower layer alternatives.
MPLS is a routine protocol that takes the most optimal path available at that given time and moves, switches and dances between different paths in real time with sub-second impact to account for changes, disruptions or down-times in any option available.
Most customers won't notice these changes, and given it improves the resilience of services for traffic to make use of all available infrastructure to reach its destination, it is a globally prevalent technology choice for carriers. But, it presents some problems in the case of transporting real time, mission critical data streams;
• It can result in higher latency because the latency paths available are variable. This is not good news when your trading currency is absolutely dependent on the speed at which it can transport. It also means that customers don't ever actually know where their data is traversing.
• A brief impact of a MPLS based protection mechanism making route changes can still carry through to fault intolerant real-time data streams (such as trading data).
• It drastically increases the complexity of isolating faults and understanding what has caused an impact to the traffic traversing the network.
Here’s How the FourNet QuantConnect Works
Normally, from a traditional carrier, organisations would have their point-to-point services built as Ethernet protected by their carriers' MPLS backbone. This achieves a high degree of resilience and redundancy, and also benefits from the performance monitoring and other operations and management capabilities that Ethernet brings over lower layer alternatives.
MPLS is a routine protocol that takes the most optimal path available at that given time and moves, switches and dances between different paths in real time with sub-second impact to account for changes, disruptions or downtimes in any option available.
- We can predetermine and openly explain to our customer 4 that in normal conditions, they can expect X. If there is a problem, their identified, chosen and custom built second route will be used, delivering them Y.
- This means that they will always know what the latency will 5 be, in any
given circumstance. - Importantly, it also enables us to immediately and accurately identify where on any path a problem occurs. It can only be on one of 2 paths, and we have full visibility on them, end-to-end, at the customers traffic layer.
- Customers can tailor their failover thresholds and parameters – when does path 2 initiate? How long do we wait to restore to path 1? It is their choice. We may even find that path 2 consistently outperforms the primary, so we can promote it and work on the other.
- We can provide proactive notice to our customers when we notice a change to latency.
Further Reading
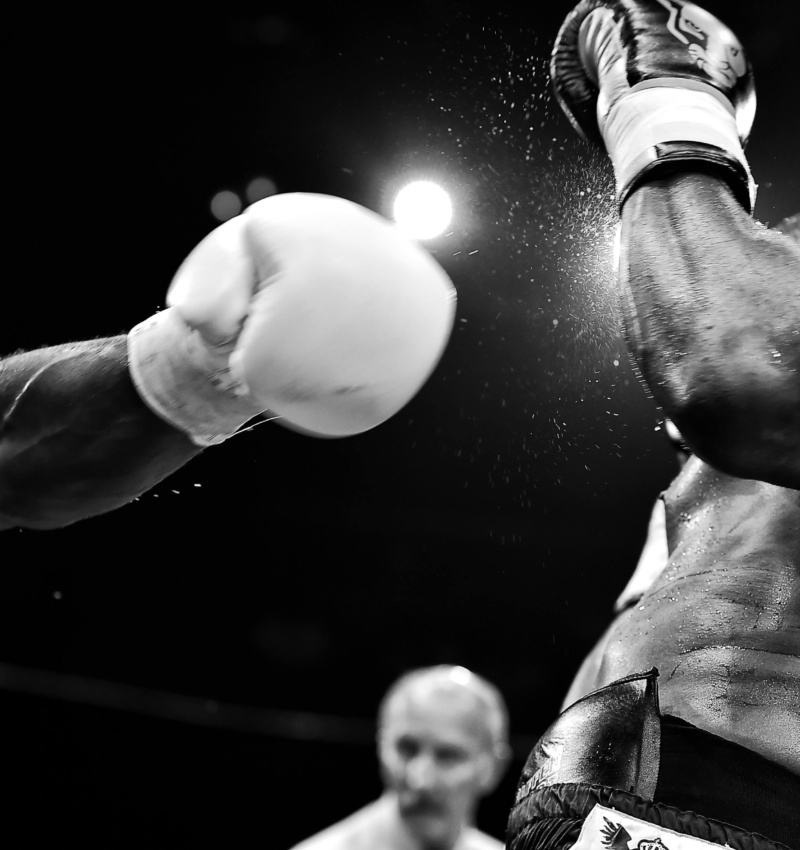
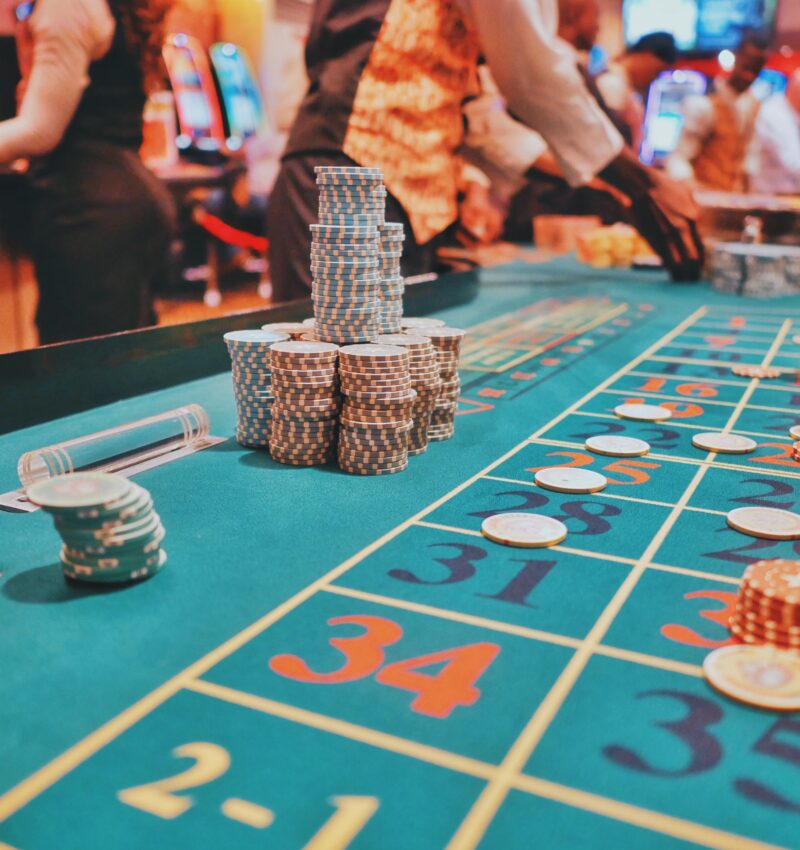
The Global Innovator of Choice for Tailored Live Casino Experiences
Secure Infrastructure
Find out MoreNavigating the Unpredictable: Security, Innovation and Leadership in a Digital World
Find out More