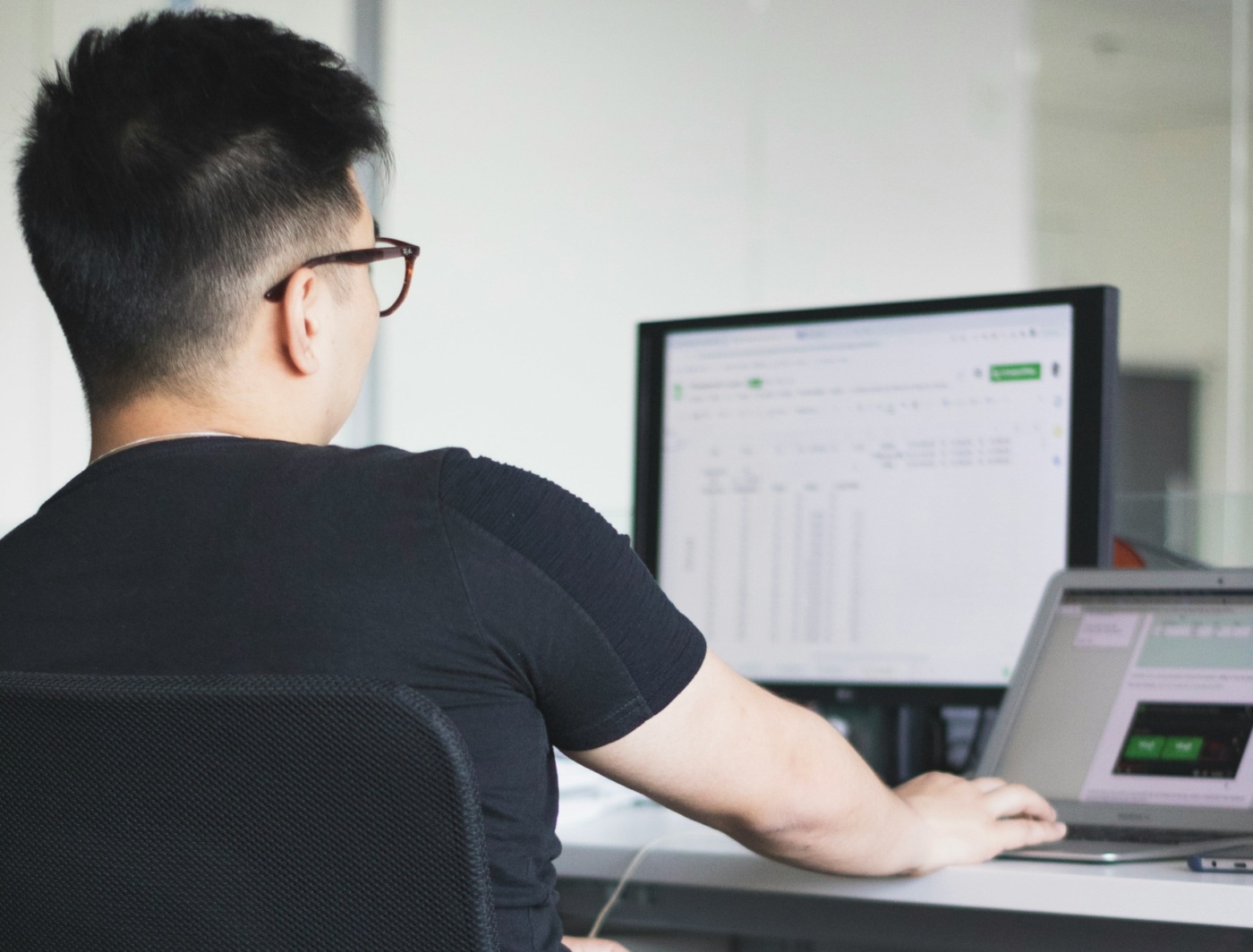
Getting the Most Out of Contact Centre Data
We believe that informed decisions stem from thorough data analysis, eliminating guesswork and assumptions. This guide explores the power of data in contact centres, covering hindsight, insight, foresight, decision-making, data quality, and the role of Artificial Intelligence (AI).
The Role of Data in the Contact Centre
Contact centres are flooded with data. Whether its insights on Average Speed of Answer, all the way to Customer Sentiment Shift Over Call Duration. Having access to all this data is great, but in our experience, many teams don't have the tools or expertise to utilise it.
Sandip Patel, Senior CX Insight Analyst at FourNet breaks down in the clip above how we group our data analysis approach into three groups:
- Hindsight involves analysing past events using metrics like call volumes, wait times, and customer feedback. For example, if customer satisfaction scores drop, hindsight data can pinpoint when the decline began, setting the stage for deeper analysis.
- Insight delves into the reasons behind these events, uncovering patterns and root causes. For instance, if a new call routing system coincides with decreased satisfaction, insight might reveal that frequent transfers are causing customer frustration.
- Foresight uses this understanding to predict future trends, enabling proactive strategies. For example, anticipating increased call volumes during a holiday sale allows for appropriate staffing, reducing wait times and enhancing customer experience.
By integrating hindsight, insight, and foresight, contact centres can transition from reactive to proactive operations, leading to improved customer satisfaction and operational efficiency.
Using Data to Make Better Decisions
While intuition has its place, data-driven decision-making is essential in modern contact centres. Relying solely on gut feelings can result in outdated practices and missed opportunities, especially in dynamic environments; but it's important to mention that data analysis and personal experience should work hand in hand. The best decisions come from a balance of data insights and human judgement with data providing the evidence, while experience adds the context needed to interpret and act on it effectively.
For example, if you’re using historical staffing patterns without checking current demand, you might miss sudden spikes in customer contact, like when a system update causes unexpected confusion, or when a new service launches and prompts a wave of questions. Real-time data helps you react in the moment, shifting resources or triggering automated support before problems escalate. Without it, you're more likely to rely on outdated assumptions that leave customers waiting and agents overwhelmed.
Data provides an objective lens to assess performance, combining historical data (hindsight) with real-time analytics (insight) to understand current situations and their causes. This clarity supports decisions on resource allocation, process improvements, and customer engagement strategies. Additionally, data-driven forecasts (foresight) based on historical patterns offer a solid foundation for future planning. Even when outcomes differ from expectations, data-driven justifications ensure accountability and continuous learning.
Ultimately, decisions backed by data are more robust, defensible, and likely to deliver positive results.
How to Build Effective Datasets
For example, a financial institution faced significant fines due to inaccurate data reporting stemming from outdated customer information, leading to compliance issues with anti-money laundering regulations. This situation could have been avoided with better data governance practices, including regular audits and validation checks.
Effective datasets require attention to detail. Raw data is preferable to pre-filtered reports, which can contain biases or errors. We recommend to always verify the source and accuracy of your data. Consistency is key, so standardise data collection methods to ensure uniformity across different systems and teams. Documentation is crucial; understanding how metrics are calculated is essential for accurate analysis.
Sandip raises his concerns about data manipulation whenever we start a new project, as existing and pre-built reports can be manipulated (unintentionally in most cases), showing inaccurate data. For instance, Adam Clough, Practice Lead for Workforce Planning and Optimisation at FourNet discusses a client who believed they were handling 500 calls a day, but they were only counting answered calls, missing an additional 500 unanswered calls. This misunderstanding affected staffing and performance metrics. Being cautious of reports designed for specific narratives is important; always seek to understand the underlying data rather than accepting summaries at face value.
By focusing on these principles, contact centres can create datasets that accurately reflect operational realities and support informed decision-making.
The Keys to Using Data
Possessing data is one thing; using it effectively is another. The key lies in transforming raw data into actionable insights that drive performance improvements.
For example, a global eCommerce company struggled with high cart abandonment rates. By analysing customer journey data, they discovered that a complicated checkout process was the culprit. Simplifying the process led to a significant increase in completed purchases, illustrating the importance of operational relevance.
Accuracy is more valuable than volume. It’s better to have a few reliable data points than an overwhelming volume of inconsistent information. Manual data handling is prone to errors, so automating data collection and reporting processes improves accuracy, reduces workload, and ensures consistency.
Continuous improvement is essential. Regularly review and refine data processes. As operations evolve, so should the methods of data collection, analysis, and utilisation. As Adam puts it, effective data usage isn’t about having the most data, it’s about having the right data and knowing how to apply it.
Preparing Data for AI
Artificial Intelligence (AI) is transforming the landscape of contact centres, but its effectiveness is entirely dependent on the quality of the data it processes. As AI becomes an integral part of business strategies, it's crucial to ensure that the data feeding these systems is structured, accurate, and relevant.
Many organisations face the challenge of having vast amounts of data without the clarity on how to access or utilise it effectively. As highlighted by our team, the first step in AI implementation is understanding what data is available and how it can be leveraged. If the right data isn't accessible or properly structured, any AI deployment is bound to fall short.
It’s also essential to recognise that AI systems have limitations; they can't solve everything. AI excels in automating specific contact centre tasks like handling web chat or processing basic inquiries, but it's important not to expect it to completely replace human involvement. For example, while AI can automate a large volume of customer interactions, it cannot always manage complex queries that require human intervention. This is where human oversight becomes vital, as AI systems must be tailored to handle appropriate tasks, with a clear understanding of their limitations.
AI systems rely on data to make decisions, and if that data is incomplete, outdated, or inaccurate, the AI will produce flawed outcomes. Ensuring data consistency, accuracy, and timeliness is critical for AI to function optimally. One way to prepare data for AI is by implementing strong data governance practices. Regular audits, updates, and validation checks can ensure that the data feeding your AI systems is high quality.
Moreover, AI is not a one-size-fits-all solution. It's essential to be strategic in identifying which areas of the contact centre will benefit most from AI automation. For instance, a natural language processing (NLP) bot can significantly improve the efficiency of a contact centre by providing 24/7 service for routine queries. However, the complexity of certain tasks might still require human agents. By being selective and strategic about where AI is implemented, businesses can optimise its value.
Ultimately, the success of AI in the contact centre hinges on both data quality and understanding the role AI can play. By ensuring data is ready for AI, organisations can create a powerful synergy that enhances customer service, boosts operational efficiency, and delivers a superior customer experience.
Related Resources
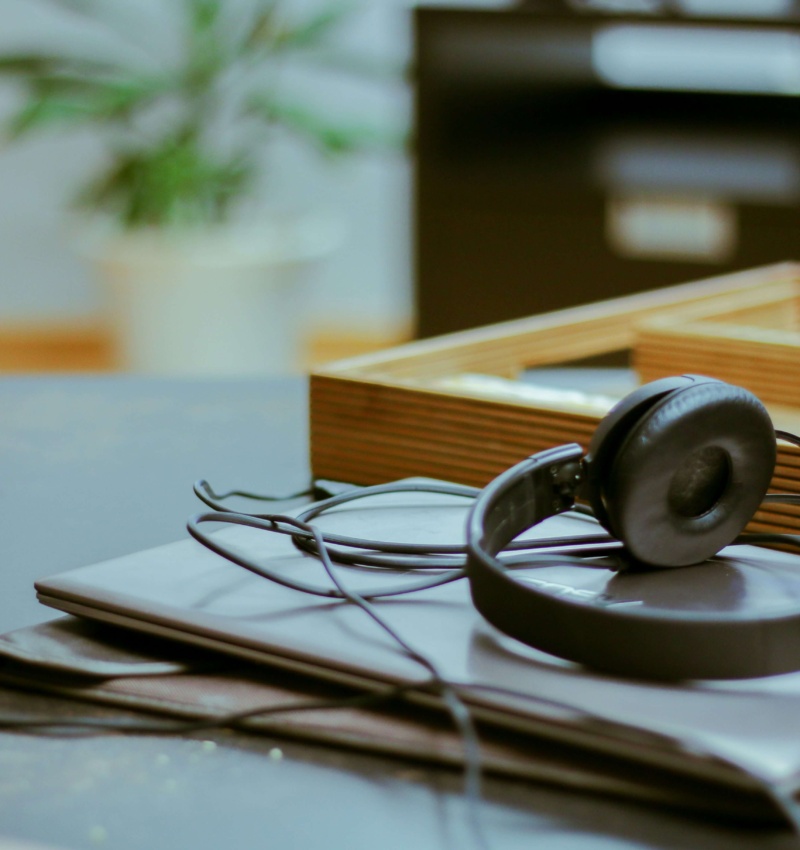
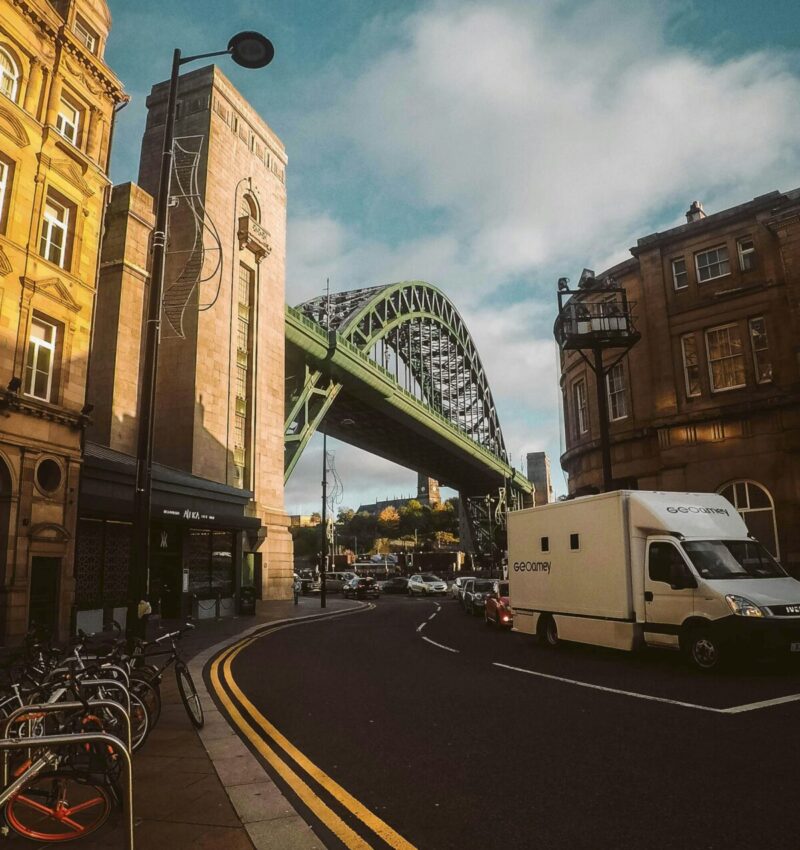
Newcastle City Council’s Journey Toward Contact Centre Transformation
Housing
customer experience
Find out More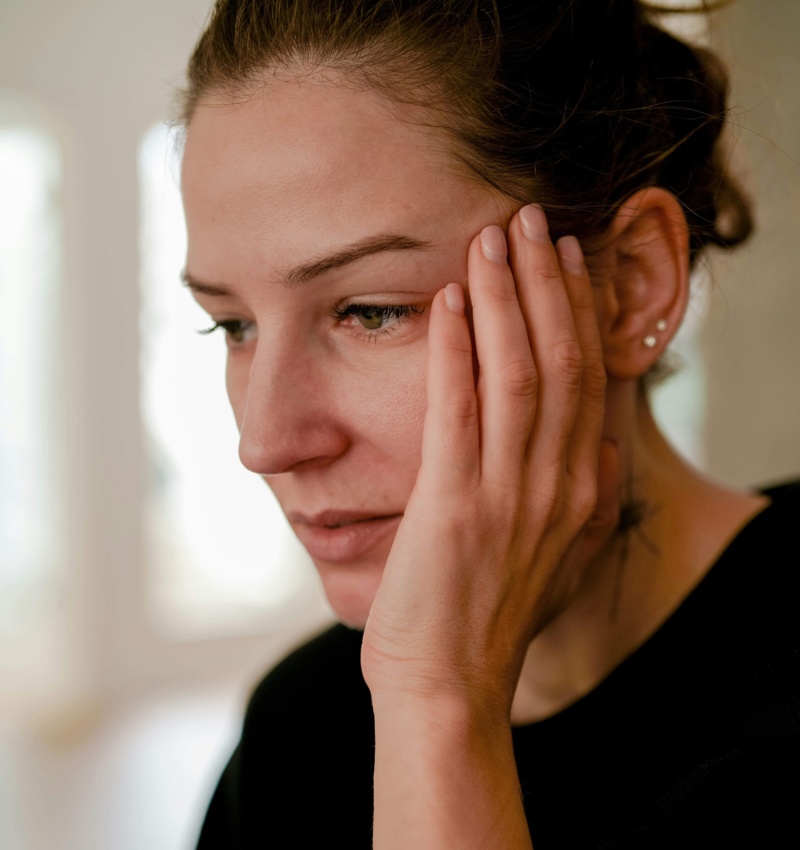